On September 5th, 2023, University of Science and Technology of Hanoi (USTH) held a doctoral thesis defense for PhD student Nguyen Chi Cuong, majoring in Information and Communication Technology with the thesis title: “Development of Deep Learning methods using Nodule Features for Lung Nodule Detection from CT Scan Images“.
The doctoral thesis is under supervision by Dr. Tran Giang Son – Co-Director of Department of Information and Communication Technology, USTH and Prof. Jean Christophe Burie – La Rochelle University (France).
The PhD thesis defense was attended by 5 members of the Thesis Jury, including:
- Assoc. Prof. Nguyen Long Giang – Institute of Information Technology, VAST – Chairman;
- Assoc. Prof. Nguyen Viet Anh – Institute of Information Technology, VAST – Reviewer;
- Dr. Thierry Urruty – Poitiers University (France) – Reviewer;
- Assoc. Prof. Le Hoang Son – Institute of Information Technology, VNU – Member;
- Dr. Le Huu Ton – Department of Information and Communication Technology, USTH – Member, Secretary.
At the defense session, PhD student Nguyen Chi Cuong presented the research results of his thesis to the jury. In particular, lung cancer is the deadliest type of cancer globally, in both men and women. In recent years, lung cancer incidence has been showing a decreasing trend as a consequence of both individual/social behavioral and medical innovations. Screening programs via low dose computed tomography (CT) have a positive impact on early lung cancer detection and treatment. Radiologists search for lung nodules during screening as the primary manifestation of lung cancer. However, screening using CT images is a non-trivial task due to the complexity of the images and the wide variety of characteristics that nodules can present. Computer-aided diagnosis (CAD) technologies can reduce the specialist’s workload by providing an independent and objective second opinion. Recently, deep learning (DL) has become the standard medical image analysis approach, but its clinical applicability is still limited. With this in mind, this thesis focuses on the development of new methods for automating lung cancer screening while considering their real-world application to local patients during the screening process.
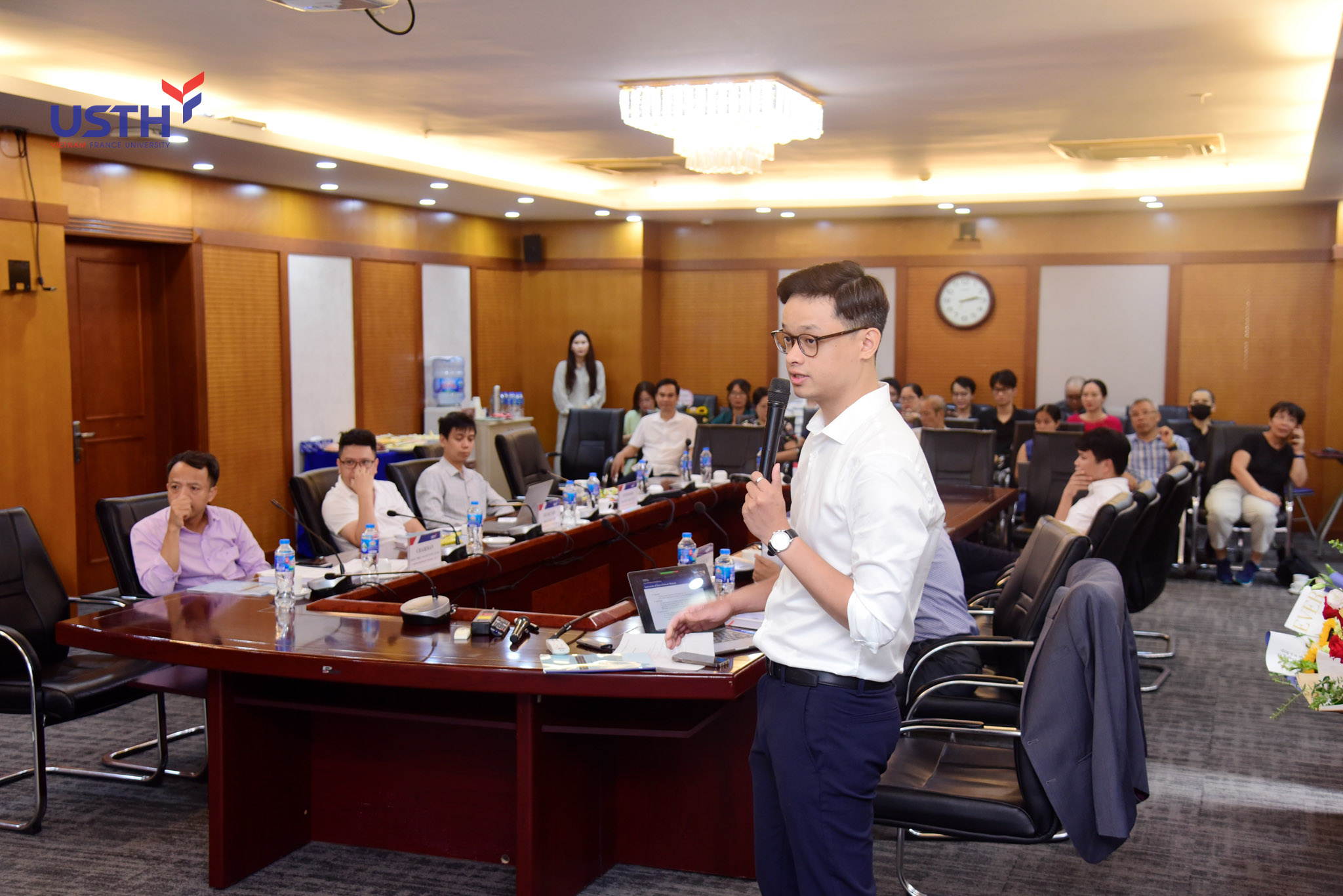
To assess state-of-the-art approaches capable of independent lung cancer screening, a study was developed to evaluate if deep learning-based methods could reliably give a chest CT assessment. The framework comprises different object detection approaches, such as the nodule detection stage that detects pulmonary nodules, followed by a segmentation algorithm. The system achieves high sensitivity, but it is still not robust enough for stand-alone operation because there are many false-positive nodules at the final prediction.
The subsequent research concentrated on developing a data-centric approach in a collaborative model of clinical experts in response to the needs of radiologists and the findings of the aforementioned study. With this in mind, a study assessing the potential of using new knowledge from a new lung cancer dataset for integrating automatic detection systems in the clinical practice of lung cancer screening was performed. For that purpose, two radiologists implemented an annotation of 372 CT scans to collect 170 nodules of sizes between 3 mm and 30 mm, called the K dataset. An automatic lung nodule detection system was evaluated using customized state-of-the-art object detection methods as a CAD system. The results show that the overall detection performance of these detection models can be impressively improved with the use of additional data from the K dataset. Our automatic detectors with customized anchor boxes based on nodule sizes and aspect ratios still significantly improve detection sensitivity without increasing the number of false positives.
Finally, the knowledge acquired with these automatic lung cancer screening approaches was used to improve small pulmonary nodule detection and reduce the false positive rate. A novel lung nodule detection approach was first developed using Faster R-CNN-based with an adaptive anchor box algorithm due to the various nodule sizes in the training data. This method generates anchor boxes from different scales and aspect ratios of ground-truth nodule sizes in the training dataset. Second, the adjacent slice method and data augmentation were used as a damethodsta enhancement method that could significantly generate new samples. In the FPR stage, a residual convolutional neural network based on ResNet architecture improves prediction quality by reducing FP rate with the trade-off of decreasing some sensitivity. Experiments show that our proposed system achieves a state-of-the-art sensitivity at very low false positives per scan.
The Thesis Jury highly appreciated the research of PhD student Nguyen Chi Cuong, which is a serious, elaborate scientific work with scientific and practical significance. The dissertation committee met in secret and voted, with a result of 5/5 votes in favor.
Before ending the defense session, PhD student Nguyen Chi Cuong gave a speech and expressed his gratitude to the teachers and staff of USTH, the instructors, friends and relatives for always agreeing and supporting for PhD students to conduct research and successfully defend their thesis.
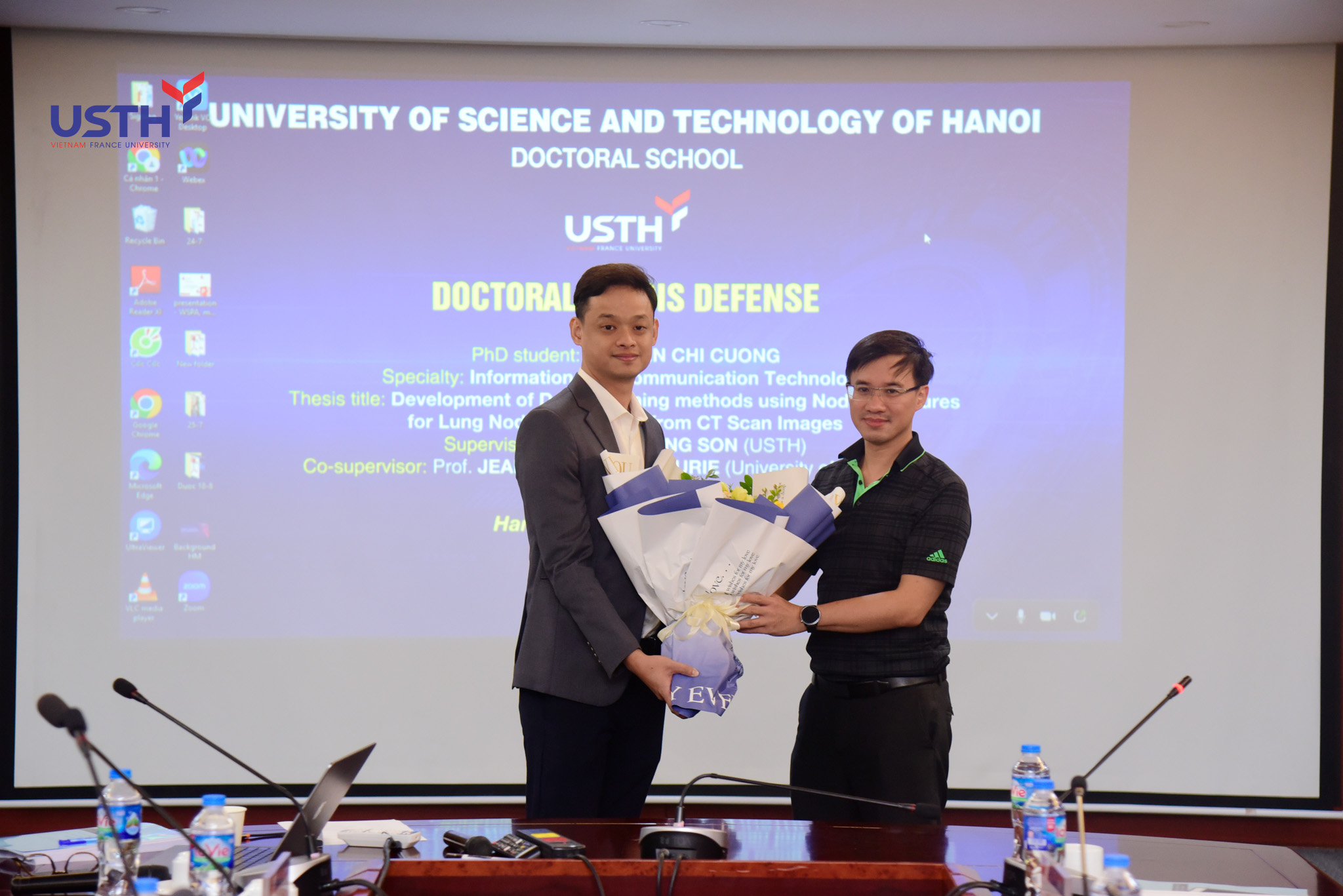
Assoc. Prof. Nguyen Hai Dang – Vice Rector of USTH sent congratulations to PhD student Nguyen Chi Cuong for successfully defending his thesis and thanked Prof. Jean Christophe Burie – La Rochelle University (France) and Dr. Tran Giang Son – Co-Director of Department of Information and Communication Technology (USTH) for enthusiastically guiding and supporting PhD student Nguyen Chi Cuong.
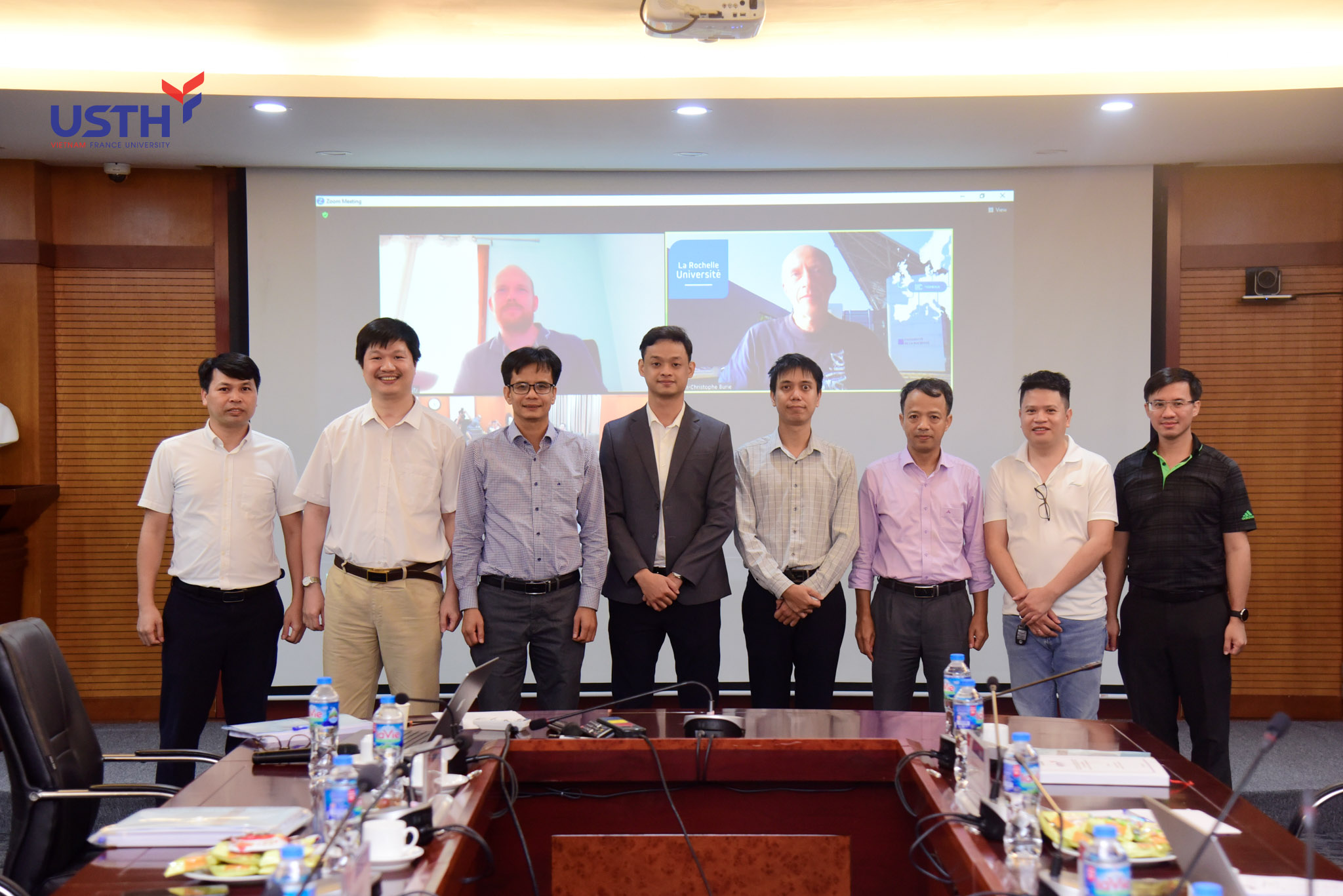
Congratulations to PhD student Nguyen Chi Cuong and wish you continued success on your upcoming path.
Some more photos of the doctoral thesis defense: